20 May 2018
We are pleased to give a keynote at the Anime Expo conference in Los Angeles, on July 5!
AI has given rise to AlphaGo and self-driving cars. What about anime? Using deep learning, we can automatically generate the perfect waifu (or husbando) for you, or prioritize your watchlist. Join us for a showcase of amazing research including manga style transfer, automatic colorization, and more!
And here is the current line-up of speakers:
Create Anime Characters using AI
We all love anime characters and are tempted to create our own, but most of us cannot do that because we are not professional artists. AI comes to rescue: on MakeGirlsMoe, you can just specify attributes (such as blonde/twin tailed/smiling) and our deep neural network will generate automatically an anime character at a professional level of quality! Our recent research is targeting style transfer from IRL pictures to manga characters.
Make.Girls.Moe got 1 million views the first 10 days. Our research proceedings were quickly sold out at the Comic Market #92 (Tokyo) in Summer 2017.
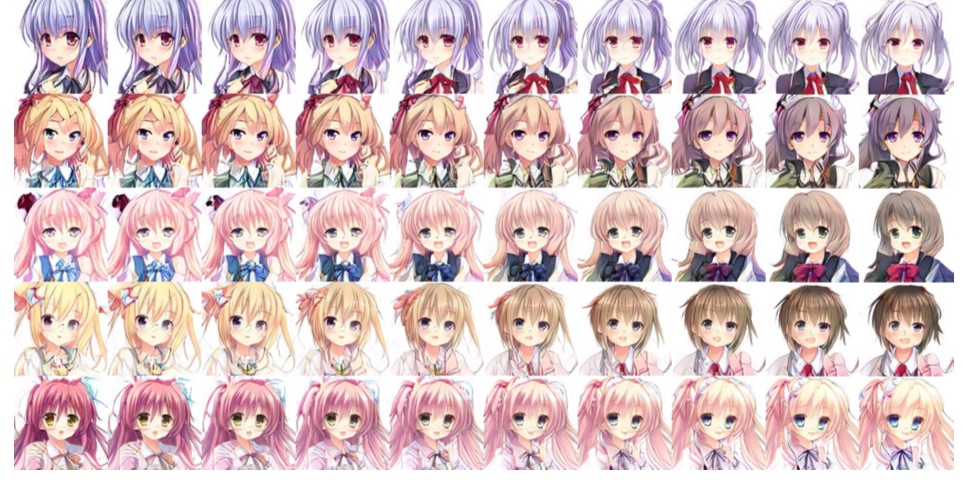
MakeGirlsMoe – Technical Report (NIPS Workshop for Creativity & Design)
Crypko – White paper
Using Posters to recommend anime and mangas
Everyone regularly ask themselves what movie, series or book they should watch next, according to their taste. Mangaki is a award-winning website that innovates access to Japanese culture through a recommender system. When a user shows up, our algorithm asks them to rate a few works. Based on their answers, they receive a personalized to-watch list of anime & manga, by geometrically positioning their ratings within those collected from other users, and using deep learning to extract information from manga covers or anime posters.
Mangaki gathered 330k ratings from 2,000 people over 11,000 anime & manga works. In Summer 2017, we released them for a data challenge organized by Kyoto University that attracted 31 submissions from 11 countries. Mangaki was awarded the first prize by the Japan Foundation (Paris branch), and an open source award by Microsoft Ventures.
Tool: Illustration2Vec by Yusuke Matsui
Mangaki – Press release – Technical report: the BALSE algorithm
Automatic Manga Colorization
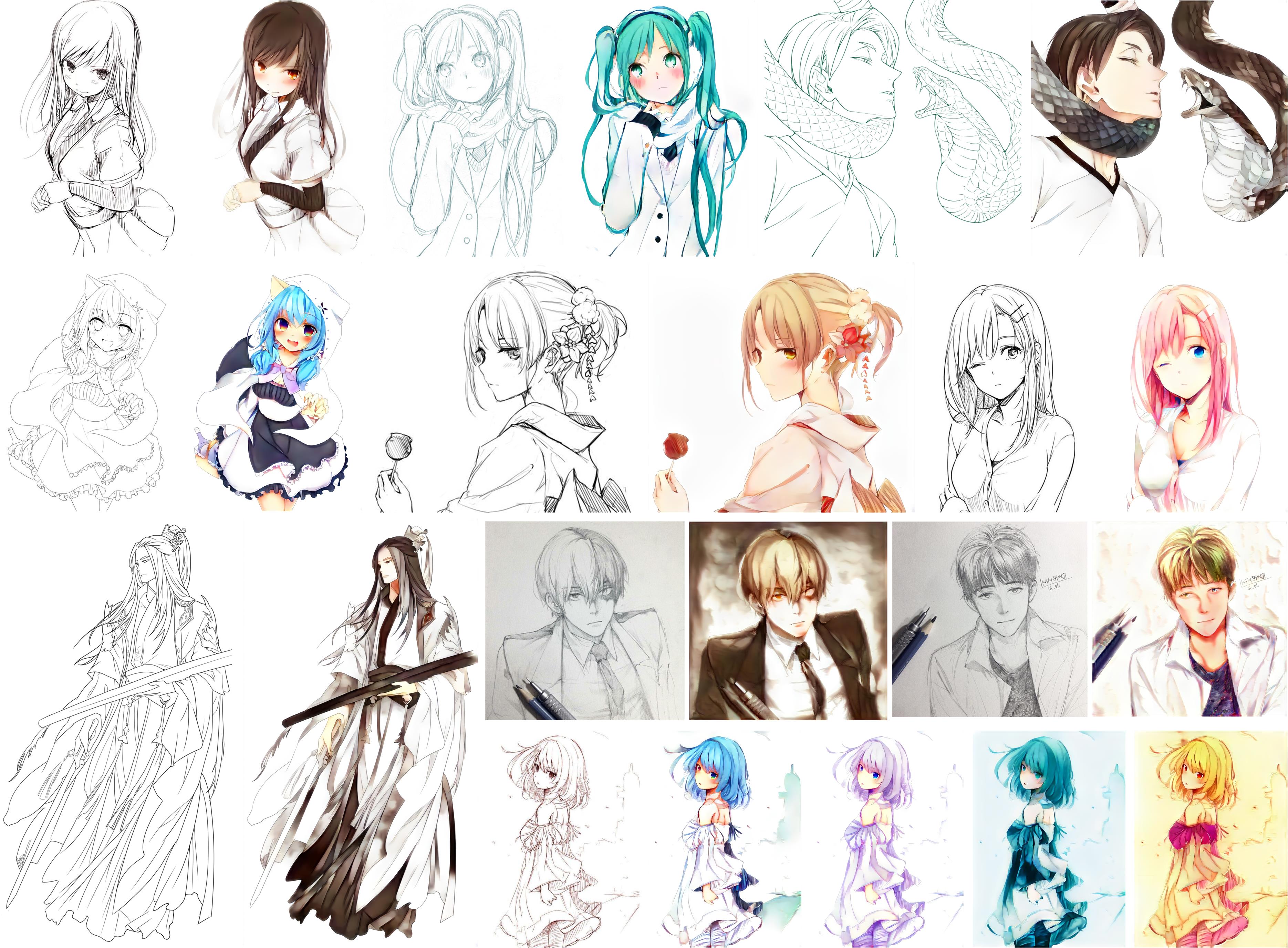
PaintsTransfer + GitHub
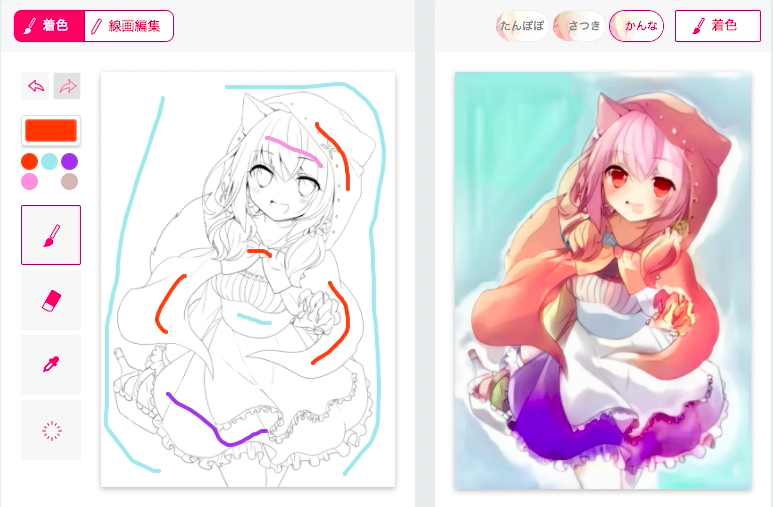
PaintsChainer by PFN
Manga Style Transfer
Cross-Domain Translation of Human Portraits.
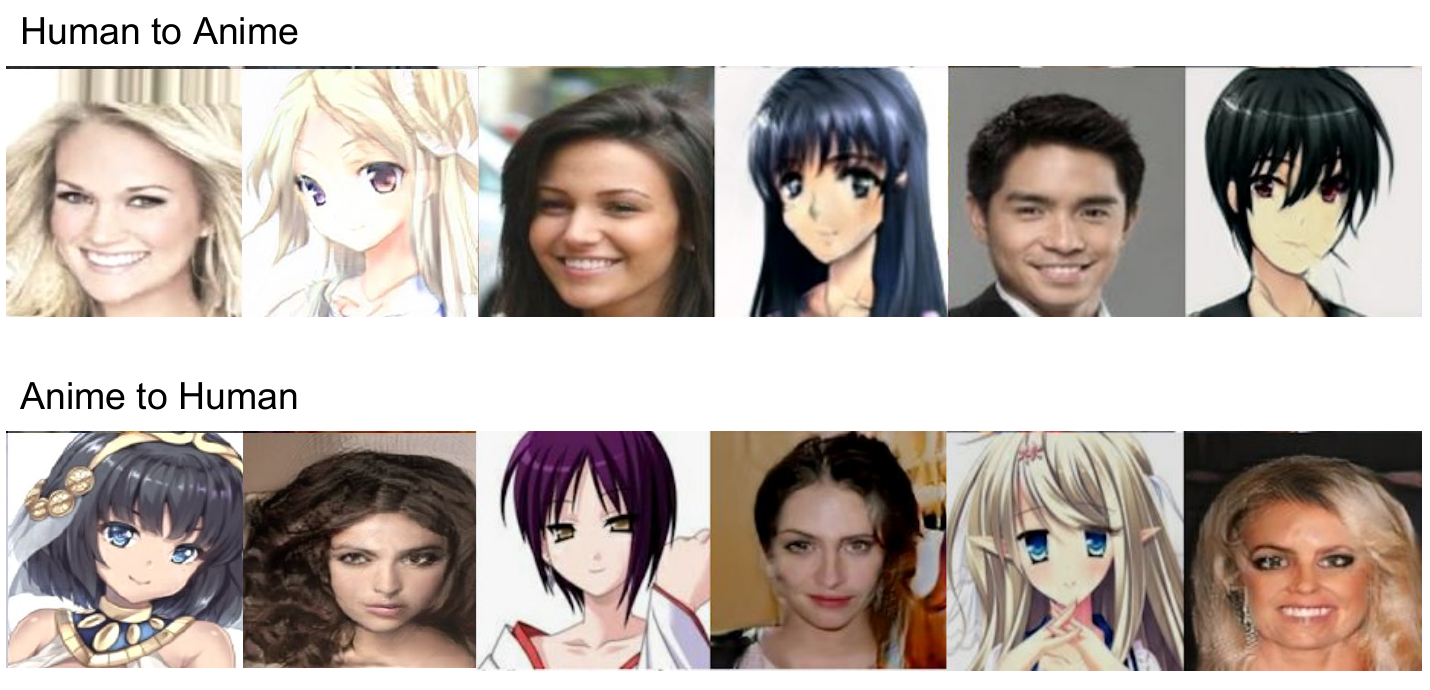
Slide from Yanghua Jin’s presentation Creating Anime Characters with GAN at the Tokyo Deep Learning Workshop held in RIKEN AIP on March 21, 2018.
Blog post about TwinGAN
Don’t miss it!
08 Oct 2017
(Cet article est aussi disponible en français.)
The Mangaki Data Challenge
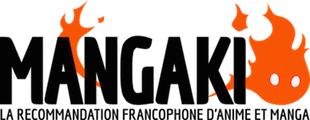
From July 1 to October 1, Mangaki and the Kashima Lab of Kyoto University organized the Mangaki Data Challenge.
The contest was announced at Anime Expo on July 2, 2017, in Los Angeles!
Statement
Participants had to determine whether certain users are interested or not in some anime or manga. Competitors had access to Mangaki ratings as open data.
Read the full problem statement in French, English or Japanese.
Leaderboard
- 1st prize.
-
GeniusIke (Microsoft, China), who wins a background artbook Your Name. and the OST of Shaft’s Fireworks movie!
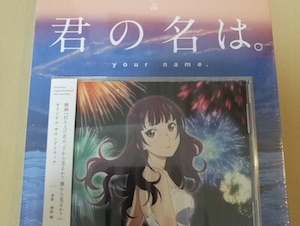
- 2nd prize.
-
ηzw, who wins a subscription to the anime streaming website Wakanim.
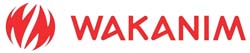
- 3rd prize.
-
karekyasu, who wins 2 collector JoJolion ecocups designed by Sedeto.
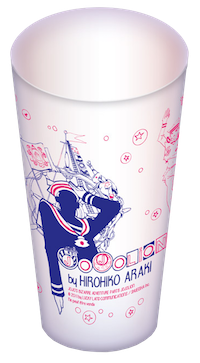
See the full leaderboard on University of Big Data.
What are the winning solutions?
The winner, GeniusIke (AUC = 86%), described his solution in a blog post and published his code on GitHub!
Please note that a simple solution that predicts a linear combination of the training set allowed BC to reach the 5th place with 82.6% AUC!
(See the leaderboard and this notebook.)
What is Mangaki’s score?
We got 81% AUC with a gradient boosting tree with which we could have been 8th. See our described solution!
We are wondering, according to our recent research article (BALSE, accepted at MANPU 2017), if using posters would have allowed us to improve this score significantly!
What countries did participate?
- France: 13
- Japan: 6
- US: 5
- China, Spain, Taiwan, Korea, Russia, India, Hungary, Mexico: 1
Why did we organize this contest?
For the following reasons:
Follow us on Twitter and Facebook to be informed of our next challenge!
And congrats again to all participants!
06 Oct 2017
(This article is also available in English.)
Le Mangaki Data Challenge
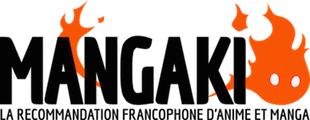
Du 1er juillet au 1er octobre, l’association Mangaki et le Kashima Lab de l’université de Kyoto ont organisé le Mangaki Data Challenge.
Le concours a été annoncé à Anime Expo le 2 juillet 2017, à Los Angeles !
Énoncé
Les participants devaient déterminer si certains utilisateurs ont envie de lire certains mangas ou s’ils n’ont pas envie de les lire, à partir du profil de tous les utilisateurs du site.
Voir l’énoncé complet en français, anglais ou japonais.
Palmarès
- 1er prix.
-
GeniusIke (Microsoft, China), qui gagne un background artbook Your Name. et l’OST du film Fireworks (Shaft) qui sortira en France le 3 janvier 2018 !
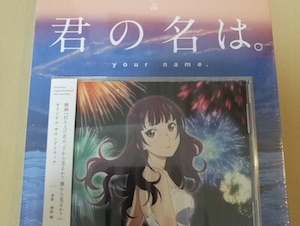
- 2e prix.
-
ηzw, qui gagne un abonnement à Wakanim.
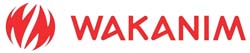
- 3e prix.
-
karekyasu, qui gagne 2 ecocups collector JoJolion par Sedeto.
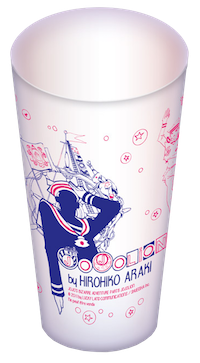
Voir le palmarès complet sur University of Big Data.
Quelles sont les solutions des gagnants ?
Le gagnant, GeniusIke (AUC = 86 %), a décrit sa solution dans un billet de blog et a publié son code sur GitHub !
À noter que la simple solution consistant à prédire une combinaison linéaire du training set a permis à BC d’arriver 5e avec une AUC de 82.6 % !
(Cf. le palmarès ainsi que ce notebook.)
Quel est le score de Mangaki ?
Une AUC de 81 % avec un gradient boosting tree qui nous aurait fait arriver 8e. Voir notre solution !
On se demande avec notre récent travail de l’été (BALSE, accepté à MANPU 2017) si utiliser les posters aurait permis d’améliorer ce score significativement !
Quels pays ont participé ?
- France : 13
- Japon : 6
- US : 5
- Chine, Espagne, Taïwan, Corée du Sud, Russie, Inde, Hongrie, Mexique : 1
Pourquoi organiser ce concours ?
Pour les raisons suivantes :
- Parler du projet open source Mangaki
- Promouvoir nos ratings en open data
(certains professeurs les utilisent déjà dans leurs cours, ou même au lycée)
- Intéresser plus de gens aux compétitions de data science
Suivez-nous sur Twitter et Facebook pour être au courant du prochain challenge !
Et encore bravo à tous les participants !
01 Sep 2017
Présentation de la nouvelle interface
Vous pouvez pour le moment suggérer une modification sur un anime ou manga depuis sa page, pour signaler des erreurs ou oublis (titre ou synopsys erroné, poster incorrect, doublon, œuvre marquée non NSFW alors qu’elle devrait l’être …).
Cependant, il était pour le moment impossible de donner votre avis sur les suggestions des autres utilisateurs : ceci est désormais de l’histoire ancienne !
Sur la page https://mangaki.fr/fix, vous pourrez ainsi voir l’ensemble des suggestions proposées par vous ou par les autres utilisateurs. Vous pourrez alors accéder à la page d’une suggestion pour donner votre avis !
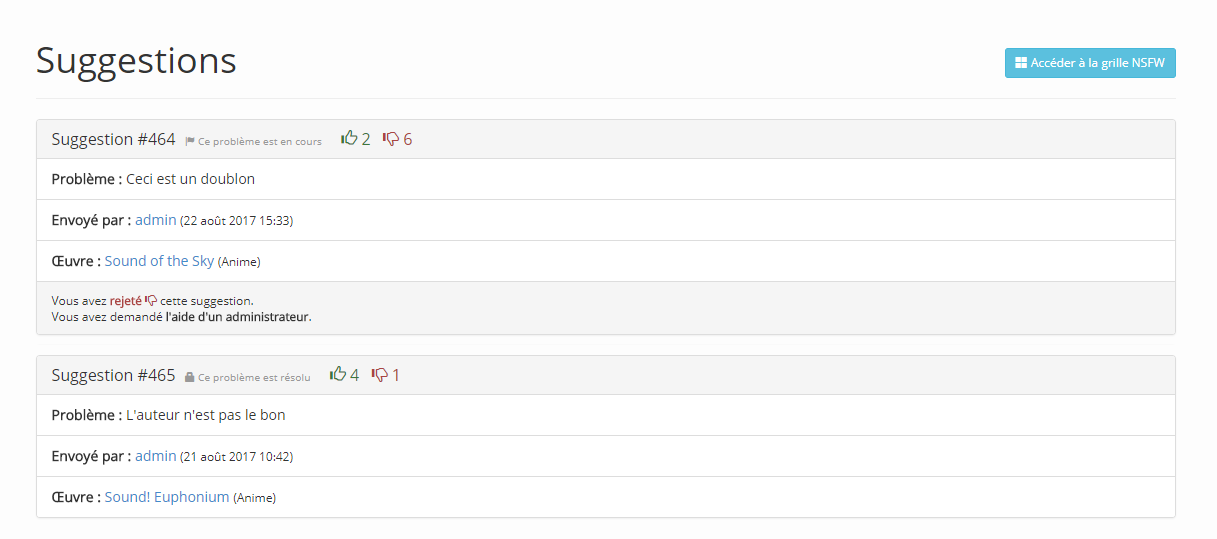
Voter sur une suggestion
En accédant à la page d’une suggestion, vous pourrez accéder à plus d’informations. Il est alors possible d’indiquer si vous êtes d’accord ou non avec cette proposition de modification. Il est aussi possible de demander l’aide d’un administrateur, pour des problèmes plus compliqués.
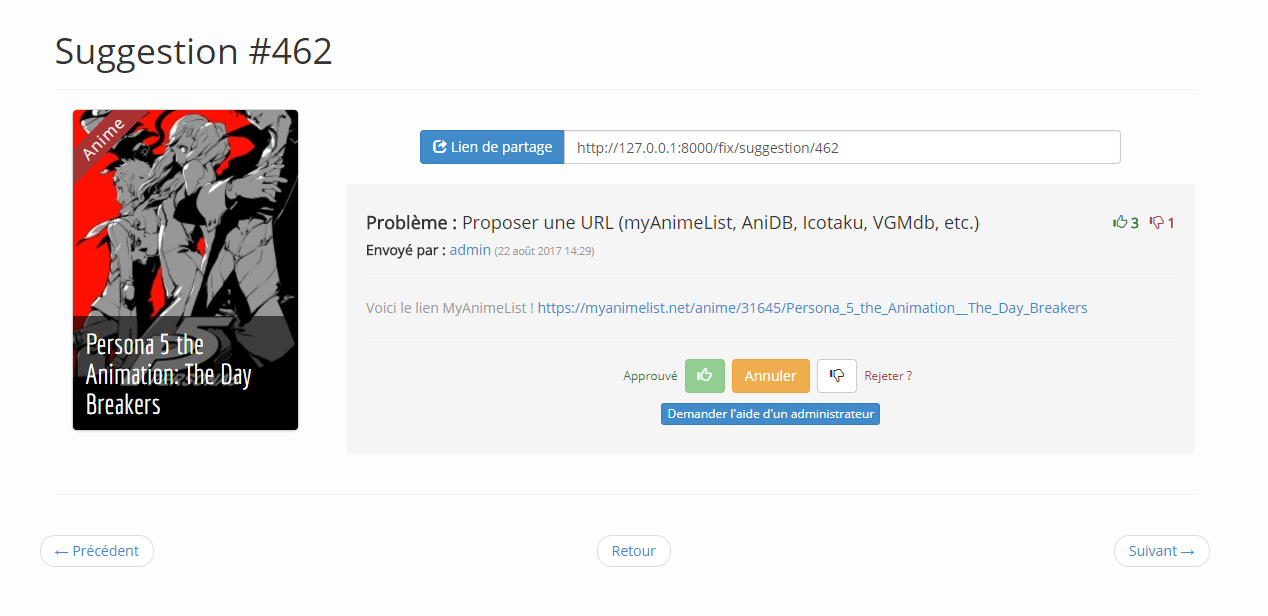
Grille NSFW
Enfin, depuis la page https://mangaki.fr/grid/nsfw, une autre nouvelle page est accessible : la grille NSFW
Sur celle-ci, vous pourrez retrouver l’ensemble des animes ou mangas pour lesquels un problème “Est NSFW” ou “N’est pas NSFW” a été ouvert. Vous pourrez alors voter pour les œuvres qui sont NSFW.
Bien évidemment, si vous avec indiqué sur votre profil ne pas souhaiter afficher les posters NSFW, il vous sera possible de temporairement les afficher sur cette grille, afin de pouvoir donner votre avis !
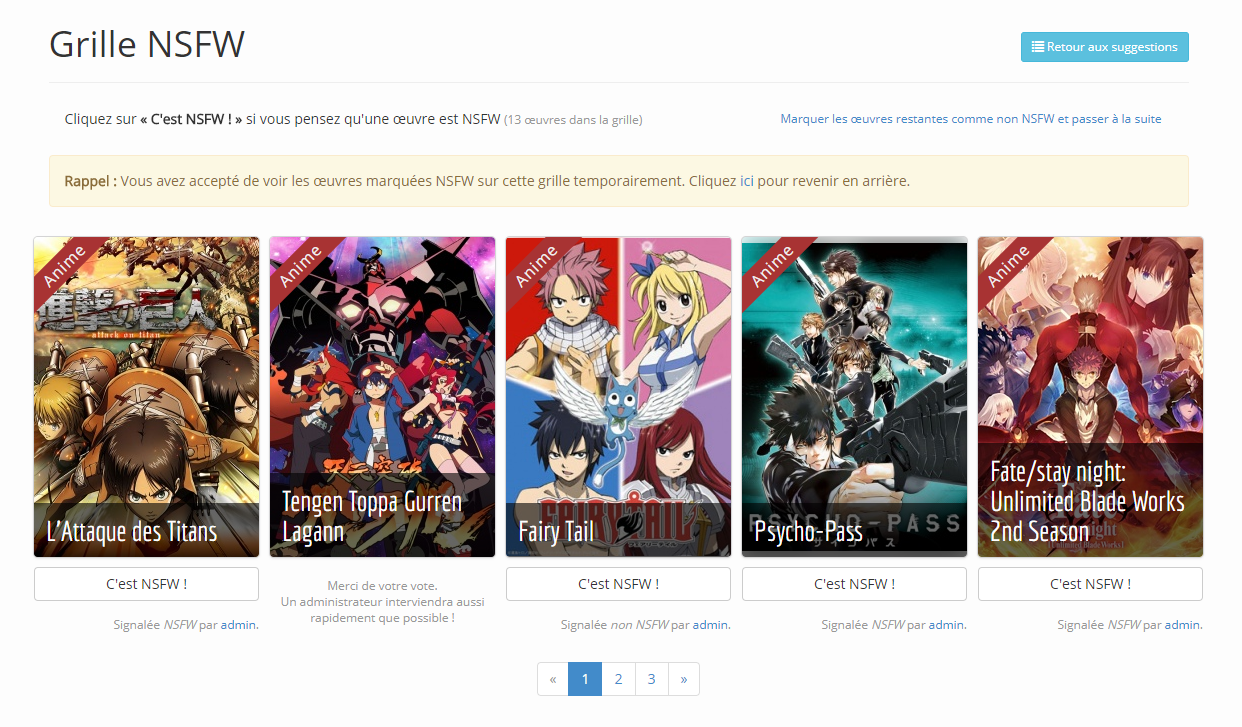
À bientôt sur https://mangaki.fr/ !
18 Jul 2017
(also in English / en français)
Mangaki.frはユーザが (1)アニメ・マンガを評価し、(2)次に見るべきアニメ・マンガの推薦を受けるためのプラットフォームです。フランスの学生により運営され、コードはGitHubで公開されています。興味がある人なら、誰でもMangakiの機械学習アルゴリズムの改善に参加することができます。
このコンペティションでは、アニメ・マンガに対するユーザの評価の予測に取り組んでもらいます。データセットとして、実際のユーザによる評価結果が提供されます。外部データの利用は自由とします。また、どのようなプログラミング言語でも参加することができます。入賞者には、手法を宣伝する機会をこのウェブページにて提供します。
アニメ・マンガの評価の種類
Mangakiでは、ユーザが「視聴後」と「視聴前」の二種類の評価を行います:
視聴後の評価には以下の4種類があります:
love
: とても気に入った
like
: 気に入った
neutral
: どちらでもない
dislike
: 気に入らなかった
視聴前の評価には以下の2種類があります:
willsee
: 見たい・読みたい
wontsee
: 見たくない・読みたくない
訓練データ:train.csv
train.csv
には以下の情報が含まれます:
user_id,work_id,rating
50,4041,0
508,1713,0
1780,7053,1
658,8853,0
1003,9401,0
...
各行は以下の情報を表します:
user_id
: ユーザID(0から1982の間)
work_id
: 作品ID(0から9896の間)
rating
: 視聴前評価。1
はwillsee
、0
はwontsee
を表す。
例えば、1780,7053,1
は、「ユーザ#1780は 作品#7053を見ていないが、その
作品を見たい(読みたい)と思っている」ことを表します。
テストデータ:test.csv
test.csv
には以下の情報が含まれます:
user_id,work_id
486,1086
1509,3296
617,1086
270,9648
459,3647
...
各行は以下の情報を表します:
user_id
: ユーザID(0から1982の間)
work_id
: 作品ID(0から9896の間)
テストデータ内のユーザID・作品IDについて、視聴前評価を予測して提出してください。具体的には、「willsee
と評価する確率」を予測し出力してください。提出ファイルの例がsubmission.csv
で示されています:
user_id,work_id,prob_willsee
486,1086,XXX
1509,3296,XXX
617,1086,XXX
270,9648,XXX
459,3647,XXX
...
XXX
に「willsee
と評価する確率」を記述してください。
ボーナスデータ1:watched.csv
watched.csv
には視聴後予測の情報が含まれています:
user_id,work_id,rating
717,8025,dislike
1106,1027,neutral
1970,3949,neutral
1685,9815,like
1703,3482,like
...
各行は以下の情報を表します:
user_id
: ユーザID(0から1982の間)
work_id
: 作品ID(0から9896の間)
rating
: 視聴後評価。love
, like
, neutral
, dislike
のいずれか。
例えば、717,8025,dislike
は、「ユーザ#717は作品#8025を見たが、その
作品を気に入らなかった」ことを表します。
チャレンジはこちら