18 Jul 2017
(also in English / 日本語)
Mangaki.fr est un site Web où les utilisateurs peuvent noter des anime et des mangas et recevoir des recommandations personnalisées (quel anime faut-il que vous regardiez absolument ?). Le site est développé par une association principalement composée d’étudiants, et tout le code de la plateforme est disponible sur GitHub, donc quiconque souhaitant améliorer le site peut contribuer librement.
Dans ce challenge, afin de gagner (et montrer votre suprématie au monde entier), vous devrez prédire les préférences d’utilisateurs sur des anime qu’ils n’ont pas vus. Les notes sont fournies par d’authentiques utilisateurs de Mangaki.
Il est plus que conseillé d’utiliser d’autres sources d’information que les données qu’on vous fournit pour améliorer votre score. Tous les langages sont acceptés, vous êtes donc libres d’utiliser celui que vous préférez ! Les gagnants pourront décrire leur solution sur University of Big Data.
Participer au Mangaki Data Challenge !
Types de notes anime/manga
Sur Mangaki, les utilisateurs peuvent noter les anime ou manga de la façon suivante :
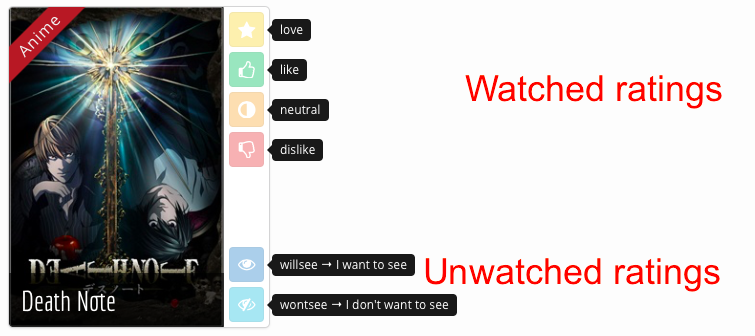
Ainsi, soit un utilisateur a vu (ou lu) une œuvre, auquel cas la note attribuée à cette œuvre est l’une de celles-ci :
love
: j’ai adoré ;
like
: j’ai aimé ;
neutral
: je n’ai ni aimé, ni pas aimé ;
dislike
: je n’ai pas aimé.
Soit l’utilisateur n’a pas vu (ou lu) une œuvre, auquel cas la note attribuée à cette œuvre est :
willsee
(ou 1
): j’ai envie de voir (ou lire);
wontsee
(ou 0
): je n’ai pas envie de voir (ou lire).
Données d’entraînement : train.csv
Le jeu de données d’entraînement, train.csv
, contient les informations suivantes :
user_id,work_id,rating
50,4041,0
508,1713,0
1780,7053,1
658,8853,0
1003,9401,0
...
où chaque ligne <user_id>,<work_id>,<rating>
contient :
user_id
: l’ID d’un utilisateur, entre 0 et 1982 ;
work_id
: l’ID d’un anime ou manga, entre 0 et 9896 ;
rating
: l’une des valeurs suivantes : 1
(willsee
) ou 0
(wontsee
).
Par exemple, 1780,7053,1
signifie que l’utilisateur no 1780 n’a pas vu l’œuvre no 7053, mais a envie de la voir.
Données d’évaluation : test.csv
Le jeu de données d’évaluation, test.csv
, contient les informations suivantes :
user_id,work_id
486,1086
1509,3296
617,1086
270,9648
459,3647
...
où chaque ligne <user_id>,<work_id>
contient :
user_id
: l’ID d’un utilisateur, entre 0 et 1982 ;
work_id
: l’ID d’une œuvre anime ou manga, entre 0 et 9896.
Pour participer, vous devrez écrire un programme qui devine les notes manquantes. Ainsi, votre programme devra produire un fichier submission.csv
de la forme suivante :
user_id,work_id,prob_willsee
486,1086,XXX
1509,3296,XXX
617,1086,XXX
270,9648,XXX
459,3647,XXX
...
où XXX
désigne la probabilité d’obtenir une note willsee
pour la paire user_id
, work_id
correspondante. Votre fichier sera utilisé pour l’évaluation.
Si vous étiez laissé dans la jungle seulement avec ces informations, le problème serait sacrément difficile. Heureusement pour vous, nous fournissons un jeu de données supplémentaire.
Jeu de données bonus : watched.csv
Le jeu de données watched.csv
contient les informations suivantes :
user_id,work_id,rating
717,8025,dislike
1106,1027,neutral
1970,3949,neutral
1685,9815,like
1703,3482,like
...
où chaque ligne <user_id>,<work_id>,<rating>
contient :
user_id
: l’ID d’un utilisateur, entre 0 et 1982 ;
work_id
: l’ID d’un anime ou manga, entre 0 et 9896 ;
rating
: l’une des valeurs suivantes : love
, like
, neutral
ou dislike
.
Par exemple, 717,8025,dislike
signifie que l’utilisateur no 717 n’a pas aimé l’œuvre no 8025.
Évaluation
Comme mesure pour départager les soumissions, le score AUC sera utilisé.
Dans le classement public, les scores intermédiaires sont calculés en utilisant 50 % du jeu de données de test, et les scores finaux seront calculé en utilisant les 50 % restants. Le classement final sera déterminé en fonction des scores finaux.
Téléchargez les données et participez au concours sur University of Big Data !
18 Jul 2017
(en français / 日本語)
Mangaki.fr is an online platform where users can provide ratings of anime and manga and receive personalized recommendations of what to watch next. It is being actively developed by a community of French crazy students, and the whole code of the platform is available on GitHub, so interested users can improve the machine learning algorithms of Mangaki.
In this challenge, in order to win (and show your supremacy to the world), you will have to predict the ratings of users over unwatched anime and manga. The ratings are provided by the actual Mangaki users.
You are more than welcome to look for extra sources of data in order to improve your score. All programming languages are allowed. Just find the one that works best for you! Winners will be provided an opportunity to promote their solutions on University of Big Data.
Compete Now!
Types of anime/manga ratings
On Mangaki, users can rate anime or manga the following way:
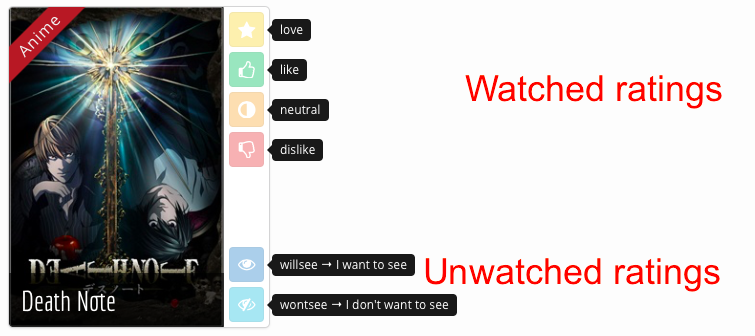
Which means either they watched (or saw) the work, in which case the rating can be one of these watched ratings:
love
: they loved it;
like
: they liked it;
neutral
: neither they liked it or disliked it;
dislike
: they did not like it.
Either they did not watch (or see) the work, in which case the rating can be one of these unwatched ratings:
willsee
(or 1
): they stated they want to watch (or read) it;
wontsee
(or 0
): they stated they do not want to watch (or read) it.
Train Dataset: train.csv
The train dataset, train.csv
, contains the following information:
user_id,work_id,rating
50,4041,0
508,1713,0
1780,7053,1
658,8853,0
1003,9401,0
...
where each line <user_id>,<work_id>,<rating>
is composed of:
user_id
: the ID of a user, between 0 and 1982;
work_id
: the ID of an anime or manga, between 0 and 9896;
rating
: one of the following values: 1
(willsee
) or 0
(wontsee
).
For example, 1780,7053,1
means that the user #1780 didn’t see work #7053, but said they want to see it.
Test Dataset: test.csv
The test dataset, test.csv
, contains the following information:
user_id,work_id
486,1086
1509,3296
617,1086
270,9648
459,3647
...
where each line <user_id>,<work_id>
is composed of:
user_id
: the ID of a user, between 0 and 1982;
work_id
: the ID of an anime or manga, between 0 and 9896.
To complete a submission, you will have to program an algorithm that guesses those missing ratings. Therefore, your program will have to output a file submission.csv
of the following form:
user_id,work_id,prob_willsee
486,1086,XXX
1509,3296,XXX
617,1086,XXX
270,9648,XXX
459,3647,XXX
...
where XXX
designates the probability of getting a willsee
rating for the corresponding pair user_id
–work_id
. Your file will be used for evaluation.
If you were left in the wilderness with this, the problem would be quite difficult. Fortunately for you, we provide a bonus dataset.
Bonus Dataset: watched.csv
The bonus dataset watched.csv
contains the following information:
user_id,work_id,rating
717,8025,dislike
1106,1027,neutral
1970,3949,neutral
1685,9815,like
1703,3482,like
...
where each line <user_id>,<work_id>,<rating>
is composed of:
user_id
: the ID of a user, between 0 and 1982;
work_id
: the ID of an anime or manga, between 0 and 9896;
rating
: one of the following values: love
, like
, neutral
or dislike
.
For example, 717,8025,dislike
means that the user #717 did not like the work #8025.
Evaluation
As a metric to rank submissions, the AUC score will be used.
In the leaderboard, the intermediate scores are calculated using 50% of the test dataset, and the final scores are calculated using the other 50%. Final ranks are determined according to the final scores.
Download the data and compete now on University of Big Data!
29 Jun 2017
1. Nouveau design des cartes
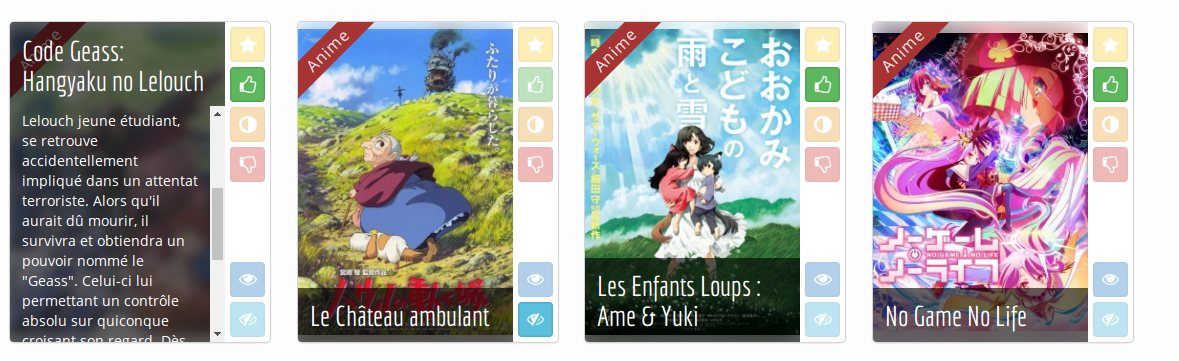
Tout d’abord, un grand merci à Elarnon qui a apporté ce design qui colore Mangaki désormais !
Ainsi, dorénavant, on ne mettra plus « en favori » une œuvre, on dira qu’on l’adore !
Cette mise à jour de design est aussi accompagnée d’une retouche pour vos smartphones et autres bidules à petits écrans.
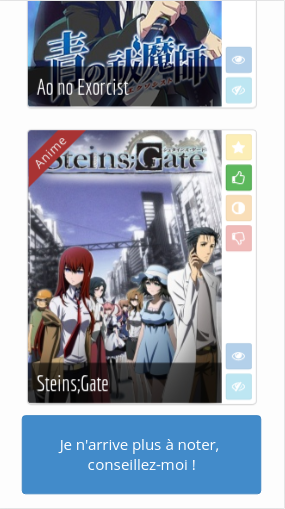
Vous remarquerez enfin ce joli ruban en haut de chacune des cartes utile lorsque vous recevez des recommandations de tous genres.
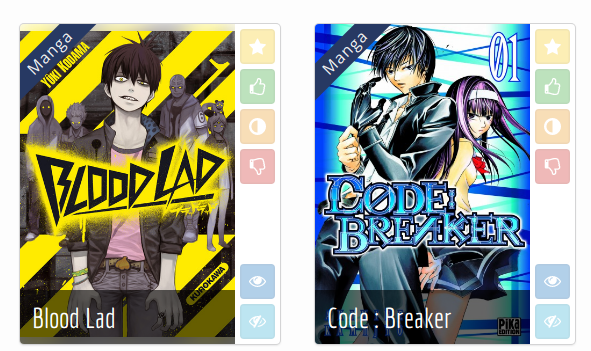
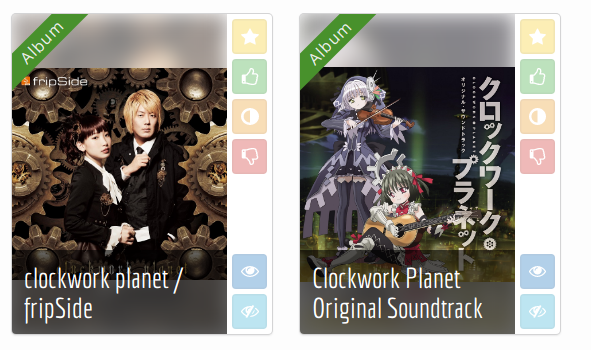
2. Tri automatique de ce qu’il reste à voir !

Un nouveau bandeau est apparu sur vos profils : en utilisant une soupe spéciale d’algorithmes, Mangaki peut vous donner dans quel ordre il faut regarder votre liste d’œuvres à lire ou voir !
En plus, vous pouvez préciser si vous souhaitez plutôt un ordre qui préfère les œuvres mainstream et les blockbusters, ou bien un ordre un peu plus inédit et exotique à la recherche d’œuvres peu connues du grand public !
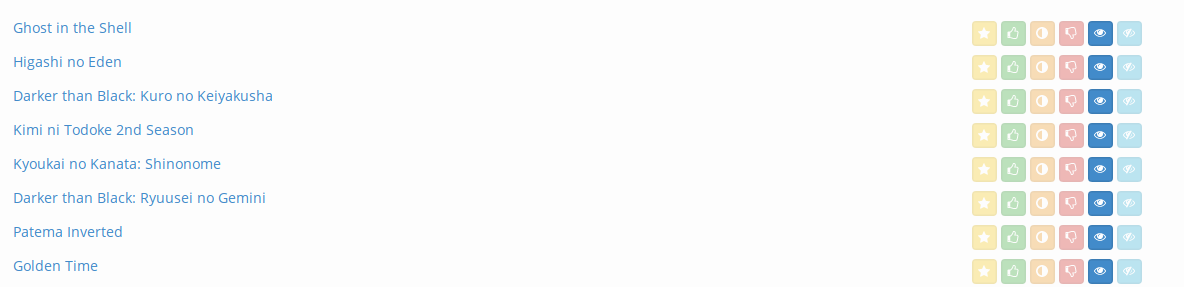

3. Nouveau design des profils
Afin de rendre vos profils plus rapides et plus simples à utiliser sur téléphone, de nouveaux onglets sont apparus.
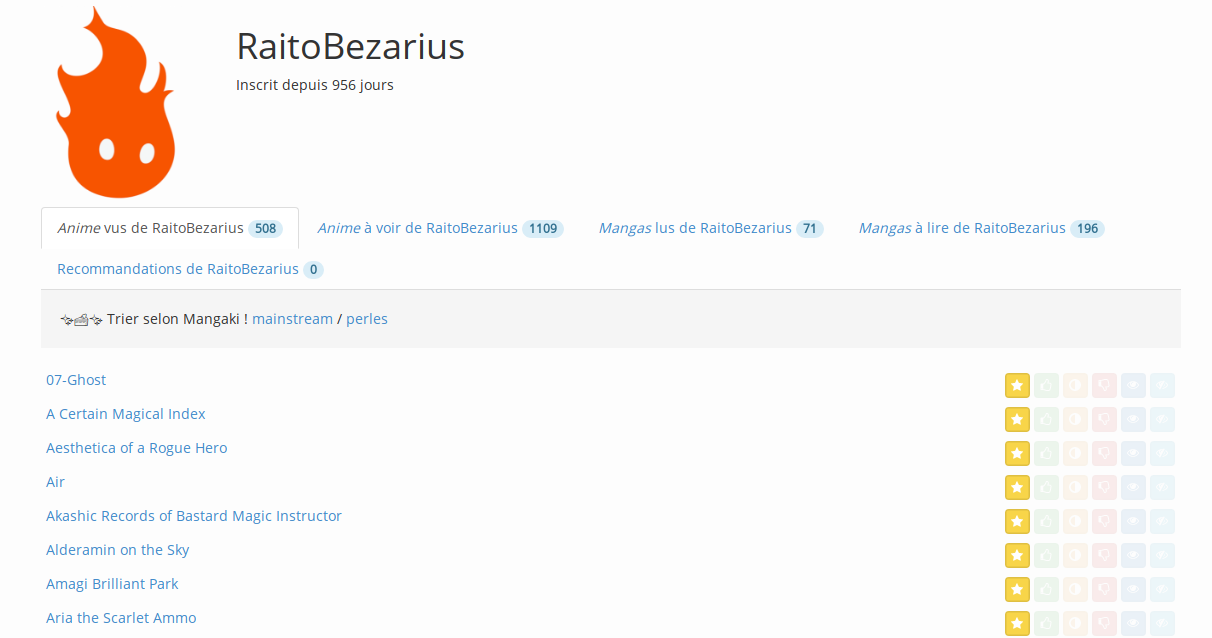
Les profils sont donc :
- plus rapides à charger.
- plus pratiques à utiliser sur mobile.
4. Essayer Mangaki avant inscription
Mais si vous lisez tout cela et que vous n’êtes toujours pas inscrit, que ça soit par flemme ou méfiance de nos recommandations, alors foncez immédiatement sur https://mangaki.fr — bonne nouvelle : plus besoin de s’inscrire !
Vous pouvez recevoir des recommandations sans être inscrit, et si vous êtes convaincus, les transférer à un nouveau compte !
Ceux qui sont déjà inscrits : vos amis n’auront plus d’excuse pour ne pas essayer Mangaki !
Bientôt dans Mangaki
- les albums : vous pourrez bientôt aimer le générique de votre anime préféré et vous faire recommander des œuvres en rapport à celui-ci !
- des synonymes : parfois on aime bien abréger le titre d’un long manga ou anime : « SnK » ou bien « NGNL ». Il sera possible d’utiliser ces synonymes pour rechercher des œuvres.
- n’avez-vous jamais déjà eu envie de mettre en attente la notation d’une œuvre recommandée ? Pour nous aussi, c’est frustrant !
- des raccourcis claviers : lorsqu’on n’a jamais utilisé myAnimeList ou qu’on a utilisé d’autres manières de suivre nos notes, il est assez fatigant de renoter tout à la souris, mais il sera bientôt possible de noter sur Mangaki entièrement au clavier !
Mais aussi bien d’autres choses telles que les nouvelles saisons d’anime et une version anglaise !
À bientôt sur https://mangaki.fr pour quelques perles !
26 Jun 2017
Mangaki organise cet été un data challenge en partenariat avec le Kashima lab à l’université de Kyoto !
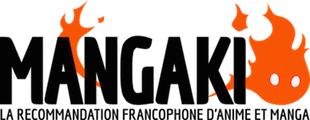
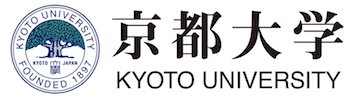
Inscriptions
C’est ici ! Jusqu’au 15 septembre 2017.
Partagez le lien : https://bit.ly/mangakidatachallenge
Sur Twitter ou
sur Facebook !
Challenge
Les participants devront déterminer si certains utilisateurs ont envie de lire certains mangas ou s’ils n’ont pas envie de les lire, à partir du profil de tous les utilisateurs du site.
Les données de Mangaki ont été évidemment anonymisées pour l’occasion.
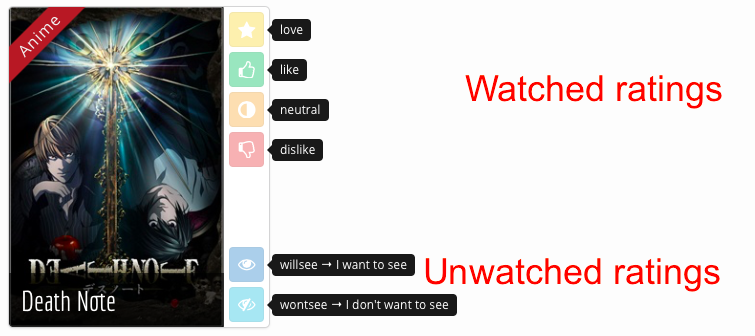
Chaque semaine, des notebooks pour aider à entrer dans les données seront postés sur notre GitHub.
Inscrivez-vous sur l’arène University of Big Data, la plateforme de compétitions de l’université de Kyoto.
27 May 2017
Mangaki va organiser un data challenge avec le labo de machine learning du professeur Kashima à l’université de Kyoto.
Les données de Mangaki seront anonymisées pour l’occasion. On publiera donc un fichier sous cette forme :
320,24,love
24,25,like
24,26,dislike
...
Bref, 350000 lignes du genre : « La personne n° 320 a adoré le manga n° 24 ».
Le principe du concours, qui sera sur la plateforme University of Big Data :
- À partir de ce fichier qui contient 80 % des données de Mangaki
- Les participants devront programmer un système de recommandation
- Celui qui prédira le mieux les 20 % restants gagnera.
On espère ainsi faire découvrir l’IA (et Mangaki) à plus d’étudiants !
Si pour une raison quelconque vous ne souhaitez pas participer à cette aventure, vous pouvez retirer votre participation depuis votre profil. Sinon, merci de nous faire confiance !